The CX Professional's Guide to Text Analysis
Text analysis is the process of deriving high-quality information from text.
In this document you will learn:
- Whether you actually need a solution in the first place
- The solution requirements and options for analyzing customer and employee feedback
- The different analysis methods: what works and what doesn’t work
- How to evalute different vendors in relation to your needs and requirements.
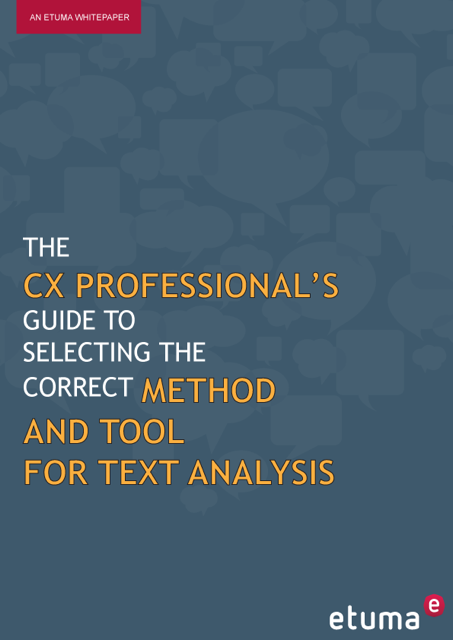
Download White Paper
Click the link to go directly to a specific step
INTRO: What is feedback text analysis and why you just might need it
STEP 1: Define the text analysis solution requirements
STEP 2: Decide how to categorize customer comments
STEP 3. Ensure that system fullfills reporting requirements
STEP 4: Create or source a categorization system
STEP 5: Choose the correct analysis method
STEP 6: Choose the text analysis tool or service vendor
Terminology
Feedback text analysis is a new industry and that’s why there isn’t a well established terminology (that everybody agrees upon). Understanding these four concepts makes the reading of this document easier.
SIGNAL: We call customer’s and employee’s open-ended text comments Signals. Some people call them open-ended comments, text feedback, unstructured feedback or verbatims.
TOPIC: Topics are what customers talk about. They try to instill the meaning of a customer’s or employee’s intention. Topics are issues, concerns and ideas that customers express in their text comments. Etuma Topics are industry specific or tuned to customer’s specification. Some people call these categories or classes.
CODEFRAME: We call a set of industry or customer specific Topics a Codeframe or Topic Codeframe. Some people call this a segment, a taxonomy, an ontology, a categorization system, or a classification system.
SENTIMENT: Sentiment is how customers feel about different aspects of your company’s operations. In Etuma’s solution Sentiment is measured at Topic level. Etuma gives a negative sentence in Signal a score of -1, neutral is 0 and positive +1. Customer’s and employee’s wishes are given a score of -0,5.
INTRO: What is customer experience text analysis and why you just might need it?
CX text analysis is a an application, which connects customer and employee voice to company’s business strategy, customer value proposition and corporate values.
Customer and employee voice is unstructured text and the volume is increasing
Loyalty management processes like NPS have increased the volume of incoming text comments, and more and more customer complaints are arriving via emails, web forms and social media. All that feedback is unstructured text.
CX text analysis makes certain business processes smarter and faster
CX text analysis runs in the background of business processes like customer complaint handling, customer loyalty management and employee engagement. It adds value by making these processes more automated, more intelligent and faster. It also saves costs by reducing slow and mistake prone human work.
LOYALTY MANAGEMENT
- Find the loyalty drivers and eaters. This enables you to move passives into promoters.
- Detect the reasons for detractor behavior. This enables you to reduce churn.
COMPLAINTS HANDLING
- Speed up complaints handling.
- More automated and efficient regulatory reporting.
EMPLOYEE ENGAGEMENT
- Retain valuable employees
- Improve hiring process
If your company is running any of these processes and the feedback volume is in the thousands per month, you need to read this document.
STEP 1: Define text analysis solution requirements
The volume of customer and employee feedback is increasing and more and more of this feedback is in the form of open-ended text comments. Naturally you need to respond to every customer complaint but what else should you do with this pile of unstructured data if anything?
1. Do you really need to analyze text?
Does your company have a customer experience strategy? Have you implemented a comprehensive customer listening system? Does your management believe that it is important to monitor every touchpoint in the customer journey? Are there one or more open-ended questions in the listening system? Is your contact center receiving complaints via email, webforms or social media services? Do you track employee commitment with a continuous employee pulse survey? If you are recognizing your company from these questions and the answer is yes to most questions, then you should continue reading.
2. What is the magnitude of your challenge?
You need to identify and list any and every tool and platform that is gathering and/or storing customer comments (Signals). This will define the magnitude of your challenge. Signals come via many channels and reside in many platforms: survey tools, customer experience management or CRM platform, social media sites, product forums, contact center platform, spontaneous emails and web-forms.
All feedback channels don’t have as valuable information. Typically your transactional Net Promoter System is the best feedback channel for extracting actionable insights. You should start by analyzing this data and then expand to other channels.
We recommend that you create a separate BI database for customer feedback. You should import all customer feedback into this customer experience database automatically (via an API) and conduct all analysis from there.
3. What is the volume of incoming text comments?
If you receive a few hundred comments a month, we recommend that you manually tabulate or classify them. If the volume gets closer to one thousand or more per month, you need to acquire an analysis tool or a service to make sense of the Signals.
4. Do you need to run customer experience text analysis in close to real-time or as periodic projects?
This depends on your industry and whether you can delay identifying problem clusters and opportunities? How fast is your industry? Do you have services that require continuous feedback monitoring?
You need to assess whether there is a need for close to real time analysis and insight extraction. How valuable is it to detect problem clusters before they escalate to other channels, like social media, in which even a small issue can inflate?
If you need to continuously monitor the customer journey then you need to acquire a CX text analysis system that learns and adapts all the time and tracks the customer pulse in close to real-time.
You can conduct periodic CX text analysis projects if your business is not so complicated: relatively few new products are launched every year, you don’t make major changes to your operations and your contact center takes care of the complaints and detects issue clusters. This turns text analytics into a periodic project rather than continuous process. For CX text analytics as a project, you might want to consider text analysis tools, in which you develop and maintain the analysis system yourself (like SPSS and SAS).
STEP 2: Decide how to categorize customer comments (Signals)
You cannot analyze customer feedback without categorizing it. This categorization has to be done systematically, relevantly and consistently. Your categorization system (Codeframe) needs to be uniform across the organization otherwise the text analysis results cannot be used in top management reporting.
Signal categorization turns open-text into statistical information, which enables you to
- Detect patterns (trends, weak signals);
- Benchmark organizational units; and
- Distribute the customer comments in real-time based on customer experience stakeholder roles.
There are four ways to categorize feedback:
1. Tabulate the feedback manually
If you get only few hundred Signals per month, this is a manageable method. With higher volumes this task becomes slow, expensive and the results are inconsistent. Humans can handle only about a dozen categories. This means that e.g. all weak signals and most emerging trends belong to the “other” category.
2. Feature in the CEM platform
Some Customer Experience Management platforms have text analysis feature. Before committing to a CEM vendor’s own text analytics, make sure that their text categorization service fulfills your quality and granularity requirements. A good way to do this is to ask for a demo. You should also check whether the CEM company has a team of linguistics experts in their staff. If not, put on extra effort into scrutinizing the categorization accuracy and relevance.
3. 3rd party embedded analytics in the CEM platform
If your CEM vendor doesn’t have a text analytics feature, then the best option is to look whether they have partners that can provide the service as an embedded analytics solution. Etuma has embedded analytics connectors for many customer insight and experience platforms like Qualtrics and Questback, CRM platforms like Salesforce and contact center platforms like Zendesk.
4. 3rd party open-ended feedback categorization service outside the CEM platform
Fourth option is to export all the open-ended comments and the relevant background variables (metadata on the Signal) into a 3rd party analysis service. We recommend that the exporting happens automatically via an API into a data warehouse or dedicated customer experience database.
Step 3. Ensure that the categorization system (Codeframe) fulfills your reporting requirements
Codeframe enables you to report verbatim analysis results in the same way as structured information (like sales figures) is reported. It creates a common language within a company and brings customer’s voice into the decision making process and has the power to transform the organization to be more customer centric.
Here are the most important categorization system requirements.
1. Encompassing
Capture all relevant words, phrases and brands from open-ended feedback.
2. Accurate
Classify those words and phrases correctly into topics and detect the sentiment for each topic mentioned. Make sure that your feedback analysis vendor categorizes a word or phrase into different topics depending on the context. A word or phrase can have different meaning depending on the context.
3. Relevant
The categorization system needs to be “tuned” into your industry otherwise you might not have the necessary granularity for different organizational departments and roles (e.g. in insurance industry you want to have all types of insurances as separate topics whereas if you are running a retail store chain, one topic called “insurance” should be enough).
4. “Whole world”
There should not be a topic called other. Make sure that the categorization system covers “whole world” (or in this case whole industry). This enables you to detect weak signals and new emerging (unexpected) topics.
5. Multi-language
This doesn’t apply to all companies. But if your customers give feedback in multiple languages, the categorization needs to be “mapped” across. This gives you the ability to view the analysis results in one language.
6. Capture brands
Most text analysis vendors aren’t necessarily able to capture your and your competitors’ brands. Make sure that the vendor you choose has the ability to capture and report brands.
STEP 4: Create or source a categorization system (Codeframe)
There are multiple ways to create the categorization system, but whatever way you choose, make sure that the system takes into account both top-down (what the management wants to see) and bottom-up (what the text makes possible) approaches. Well working categorization system requires a couple of iterations and is a balance between these two views.
Designing and implementing a uniform categorization system might seem like a daunting task but the benefits are clear. Uniformly categorized customer comments have the power to transform your organization.
1. Manually
This must be more of a top-down approach because humans can only handle about a dozen discrete categories. This method is slow and it can also be expensive in higher feedback volumes. It is more suitable for BtoB companies. It is important to notice that there will not be a “whole world” view: it will be difficult to detect weak signals. Also, trend analysis can be unreliable because of inconsistency of human tabulation.
2. Do it yourself using text analysis modelling tool
There are excellent tools like SPSS and SAS to create a model to analyze open-text. The challenge with these tools is the steep learning curve and the need to continuously tune the analysis. You need dedicated, well-trained professionals to take care of this work.
3. Use text analysis vendor’s industry specific Codeframe
A generic Codeframe will never provide accurate and relevant analysis results. The reason for this is that in different industries words might have a different meaning and thus need to be categorized differently. This is why, at minimum, the analysis results presentation needs to be industry specific. Some text analysis vendors focused on analyzing customer feedback have created a productized industry specific categorization system (Codeframe). They also continuously improve the accuracy and relevancy of these systems. These systems are usually very accurate but might not fulfill your information granularity requirements. Ask for a demo, and you will see whether your needs are fulfilled.
4. Modify text analysis vendor’s Codeframe
Text analysis vendor’s generic or even industry specific Codeframe might not fulfill your granularity and reporting requirements. Make sure that either the vendor provides modifications to the Codeframe as a service or they give you an easy-to-use tool which you can use to modify the Topics.
STEP 5: Choose the correct analysis method
There are a number of ways to analyze open-ended customer comments. Some provide statistically relevant information, others don’t.
What doesn’t work
These methods fail because they are inaccurate, don’t provide statistical information, and/or the information is too general or too granular. There are very few feedback analysis companies left in the market that follow these methods.
- Brand extraction is useful information when it comes to social media and media discussions. But it fails to provide actionable information when it comes to your own feedback data analysis: it doesn’t tell WHAT people are talking about.
- Extracting whole comment sentiment based on the number of good and bad words in the whole comment and how far are they from the brand mention is quite easy but it fails to provide information about the topic of discussion: WHAT people are talking about. It also often fails to get the sentiment right because the sentiment analysis is based on a list of good and bad words and not the structure and rules of the language.
- Extracting keywords doesn’t work because keywords cannot be turned into statistical information: there is almost an infinite number of keywords in any language. The topic of discussion is statistically distributed “too wide”.
What works
An analysis system that uses natural language processing combined with artificial intelligence ‘understands’ text the same way as us humans do.
- It maps relevant brands, words and phrases into “contextual baskets”. We call these baskets ‘topics’. Detecting the sentiment of each Topic mention increases the usability of the analysis results.
- Analysis must be industry specific. The nature of language dictates this: words and phrases can have a different meaning depending on the industry. Also the necessary analysis granularity level depends widely on the industry.
- If you are a customer experience professional working for a hotel chain, you want to be able to track all the key experiences in a hotel stay (CHECK-IN, CLEANLINESS, TOWELS, BED, SAFETY, MINI BAR, NOISE, WLAN etc.). If you track the airline customer journey, a topic called HOTELS might be sufficient.
- If you are a customer experience professional working for an insurance company, you want to know how your different products (CAR INSURANCE, TRAVEL INSURANCE, HOME INSURANCE etc.) are performing. If you are tracking the telecom customer experience, a topic called INSURANCE might be sufficient.
- Sentiment analysis must be based on language structure and rules. It is important to know how people feel about different aspects of your operations. Each topic mention should be scored. We think that three-level sentiment scoring is sufficient on topic level (NEG-NEU-POS) and five level for the whole comment (VERY NEG to VERY POS) .
STEP 6: Choose the text analysis tool or service vendor
At this point you have identified the need for a text analysis solution: Your management has a customer experience management strategy, you get enough feedback and you have a need to make sense of that feedback fast and feasibly. Now you need to choose a tool or service vendor.
Choosing the right vendor to analyze customer verbatims is a difficult task. The websites of most vendors don’t provide any kind of information on how they accomplish the feat, and finding out what concrete benefits you’ll get out of their services can seem obscure.
This list is somewhat overlapping with the previous steps but we wanted to give you clear set of requirements. It is up to you to decide how you weight different aspects.
Twelve criteria for choosing a verbatim analytics provider
But choosing the right text analysis solution is easier said than done. It is hard to justify the investment with a meaningful ROI calculation (what is the value of better information?), and choosing the right solution among the many text analysis methods and approaches can seem like a daunting task.
In his excellent blog post “12 Criteria for Choosing a Text/Social Analytics Provider”, Seth Grimes tries to make the vendor selection process easier by creating a common-sense list of requirements and checkpoints. Because Seth’s list covers the requirements for all kinds of text analysis, I will try to paraphrase it from the point-of-view of open-ended customer and employee comment analysis (verbatim analysis).
His advice–to keep a clear head and set realistic expectations–is great:
“Some preliminary advice: Work back from your business goals. Determine what sorts of indicators, insights, and guidance you’ll need. No business is going to need 98.7% sentiment analysis accuracy in 48 languages across a dozen different business domains. Be reasonable; stay away from over-detailed requirements checklists that rate options based on capabilities you’ll never use. Create search criteria that separate the essentials from the nice-to-haves and leave off the don’t-needs. Then design an evaluation that suits your situation – include proof-of-concept prototyping, if possible – to confirm whether each short-list option can transform data relevant to your business into the outputs you need, with the performance characteristics and at a cost you expect.”
1. Type of text (this is the only point I added to Seth’s list) + Industry & business function adaptation
Your verbatim analysis vendor should have a dedicated solution specially tuned for analyzing customer feedback. The way customers “speak” in their feedback comments is often very different from the text in other type of documents.
Words often have a different emphasis and meaning in different industries. For example, as mentioned earlier, a hotel chain needs to track all the key experiences in a hotel stay: CHECK-IN, CLEANLINESS, TOWELS, BED, SAFETY, MINI BAR, NOISE, WLAN etc.. For a hotel chain, a generic topic HOTELS would not provide interesting information. For another industry a topic called HOTELS might be sufficient or the topic might not be interesting at all.
Contextual understanding is crucial, which is why the analysis presentation needs to be industry specific. The text analysis provider must be able to identify and understand the context of a keyword, and classify it into topics that are structured according to a industry-specific codeframe (a.k.a ontology or categorization system).
2. Customizability
Each company has its own business targets and services. The analysis system must be able to capture your brands and map them into topics. This will give you the ability to track your brands. If you also crawl social media, make sure that the text analysis vendor can capture your competitors’ brands.
Text analysis systems are never perfect. Even if the analysis is great, the topics could be named or structured wrong. That’s why you or the vendor need to have the ability to tune the analysis and the topic codeframe. The main consideration here is who does the customization: you (or somebody in your staff), a third-party consultant, or the analysis vendor. Most tools and services expect you do that customization work. Unless your vendor provides this as a service, make sure that you have the necessary computational linguistic knowledge, and add the cost of this work into the total cost of ownership.
3. Data source suitability
Each feedback channel requires analysis tuning to accommodate the channel specific peculiarities and language. Tweets require a different kind of analysis than chat logs. For example, being able to analyze emoticons is important in social media but not required for spontaneous feedback via web form or email. Make sure that your vendor fulfills the channel specific analysis requirements.
4. Languages supported
One of the main differences between vendors is whether and how they analyze multiple languages. Translating comments loses nuance and often, in the case of smaller languages, gets the meaning (topic) and sentiment wrong. That’s why it is important to analyze the feedback in the source language.
Presenting the results can be done in a single language but only if all the topics and topic groups are “mapped” across languages. This creates comparable information across languages, and makes your headquarters happy.
5. Analysis functions provided
Text (Topic) doesn’t have an absolute benchmark, except how many times the same topic was mentioned during a time period. This is why consistent industry-specific categorization (point 2) is essential in verbatim analysis. When each topic is tagged with sentiment, then we are already talking about two-dimensional information. This makes extracting actionable insights possible.
Name or brand detection (Named Entity Recognition) is crucial, and it would be great if brand mentions could also be tagged with sentiment.
Having access to the keywords that are tagged to a topic can also be valuable but brings up the challenge of handling a large amount of less-structured data (a feedback channel can have tens or hundreds of thousands of keywords).
Check also that the vendor can break the comment into sentences and tag each sentence with a topic. Without this function, you have to read the whole comment; With it you only have to read the contextually relevant (topic specific) sentences. This makes root-cause analysis much easier.
The key is to have information that can be used for decision making. What this means in practise is techniques such as clustering (e.g. which topics often occur together), correlation (how a topic correlates with a quantifiable background variable – e.g. NPS score), and trend analysis (how topic volume or average sentiment changes over time). If you want to find out more techniques, read thisblog post.
6. Interfaces, outputs and usability
Feedback text analysis application should be offered in multiple ways but in order to be able to run it in the background an API from survey system to feedback text analysis application and then returning the analysis results to your data warehouse is a good option. The other possibility is a so called embedded analytics solution, in which the analysis runs behind a customer relationship management (CRM) or customer experience management (CEM) platform.
Verbatim analysis results are much more valuable when combined and correlated with other data (e.g. survey metadata, demographics, purchase or web behavior). Verbatim analysis visualization ‘in vacuum’ is not that interesting.
Data enrichment should be done transparently (via an API) either by enriching text analysis data or even better having text analysis results enriching data in other systems (e.g. CRM).
The verbatim analysis results should be also be usable for data mining (e.g. SPSS and SAS data analysis tools). You should use your existing dashboard platform (e.g. Tableau, Qlik, PowerBI) for the analysis results distribution. You can also use a combination of email and excel to distribute contextually relevant comments to customer experience stakeholders.
7. Accuracy: precision, recall, relevance and results granularity
If the text analysis vendor uses a combination of Natural Language Processing libraries for text analysis, artificial intelligence to map the analysis results into topics and industry specific codeframes to present the results, you should be in pretty good shape when it comes to precision and relevance.
But when it comes to granularity, you need to check out or even better run a demo and find out vendors ability to tune the analysis results (topics or categories) according to your company’s needs (=be able to extract what customers and employees are talking about strategic initiatives, customer value proposition and corporate values). If they don’t do that as a service, you need to make sure that their analysis customization tool is easy to use.
8. Performance: speed, throughput and reliability
If you are running a fast paced B2C business with continuous loyalty and employee survey processes, the verbatim analysis service should run close to real-time. What this means is that the feedback text analysis application takes no more than few seconds or minutes to analyze even a large set of customer or employee comments.
If the vendor solution runs in one of the global cloud platforms (IBM, Microsoft, Amazon) then the performance should not be an issue. If the vendor runs their own servers then you need to find out a way to test whether the vendor fulfills your throughput and response time requirements.
Running the analysis in your own servers is an option but in this case the verbatim analysis vendor cannot tune and maintain the codeframe: you need to do it. Make sure that you have the necessary competencies and enough time to allocate to this work.
9. Provider track record, market position and financial position
The sad truth is that nobody ever gets fired for buying from IBM. But not considering smaller and often more innovative (and much cheaper) service providers would be a mistake. Cloud platforms have made even the smaller vendors’ services of high quality, secure, reliable and scalable. Therefore the quality cannot be deduced from the size of the company. These cloud-based services (in which the vendor provides the analysis as a turn-key service) are often much cheaper to own than traditional enterprise software solutions.
What kind of set-up makes sense for you depends on your financial and human resources (=how much text analysis platform maintenance you want to do). But make sure that whomever you choose they have references.
The best way to ensure the quality and relevance of the analysis results is to get the vendor to run a demo with your own dataset (=familiar context). That will demonstrate whether the vendor can meet your requirements. It is good to keep in mind with demos, especially when free, that the quality is not as good as with the commercial implementation. But demo is good way to figure out whether their analysis results meets your relevance and granularity requirements. Those are often depended upon the service architecture and cannot be changed (except if you create the analysis model in a text analysis tool like SPSS yourself).
10. Provider’s alliances and tool and data integrations
If the verbatim analysis vendor provides a well productized API and you have the infrastructure (enrichment, analytics and visualization tools) then it doesn’t really matter what kind of alliances they have. You can get the text comments analyzed via an API and get the analysis results back to your customer experience database.
But if you want to integrate your feedback channels directly to the verbatim analysis application and then visualize the results in the CEM or CRM platform, then the process is probably easier and more cost-effective.
11. Cost: Price, licensing terms, and total cost of ownership (TCO)
There is a large TCO difference between a pure cloud based service and a platform that you need to maintain and tune. It might be hard to figure out whether the vendor provides a platform or a service. A demo or proof-of-concept will demonstrate this better than thousand powerpoint slides. You should also check what is the minimum contract term. If the vendor requires more than one year lock-in then you need to conduct some additional due diligence.
12. Proof of concept
The fact that the vendor cannot provide a free demo with your data tells a lot about the service. If they don’t provide a free demo, it means that their solution architecture requires so much tuning and customization that developing a demo isn’t feasible. What is important to keep in mind is that this type of solutions are also more expensive and the maintenance costs can be substantial because they are customized to each customer separately. But typically the analysis accuracy and relevancy in this type of solutions is good.
If the demo is giving you an access to a text analytics platform/tool (e.g. SPSS) to try out for 30 days, then you really need to make sure that you have time to develop the analysis model during that time. This type of tools are excellent but only if you have the knowledge and experience, and a lot of time to allocate to this effort. The learning curve can also be steep and you do need to have at least a basic understanding of linguistics.
If you are not familiar with text analysis tools and don’t have a large budget then analysis-as-a-service (AaaS) is your best option. The nice thing about these type of cloud services is also that they are easy to implement. It is also important to keep in mind that because the investment is relatively small, you are not tying yourself to a single vendor forever.
Now that the verbatim analysis business has gone mainstream and there are many solutions which are developing at different speeds, you just might want to keep an option to change vendors even in the near future.
Seth’s advice about working back from business goals is very valid. Decide the business target (what information, granularity, multi-language, real-time) and take the restricting elements (budget, linguistics knowledge, resources) into account, and you will end up making the right decision. Here is a link to a web page that lists 62 text analysis companies. Follow Seth’s and my advice and you will end up with a solution that makes your job easier and your work more valuable for your company.